Granular modelling allows us to make far better predictions.
When estimating the value of a portfolio we may choose to adopt an aggregate approach – looking at specific segments and treating each of them as a whole – or a granular approach – estimating the potential value of each account or customer individually and aggregating at the end. The latter is more complex both computationally and in terms of methodology, but it has significant advantages in flexibility, accuracy and reusability.
Granular modelling is flexible because it makes no assumptions about the specific segments we are interested in. Its results may be aggregated based on any available characteristics, and so compared or made to conform to any desired segmentation approach. This is especially important when we are forced to adopt alternative segmentations due to lack of appropriate data.
Granular modelling is accurate because it exploits the full breadth of information available without making assumptions about the characteristics of specific segments. When the granular approach is based on data-driven statistical modelling, meaningful predictors will be chosen automatically, regardless of segment membership, and any ‘noise’ due to bad data quality is still averaged out when looking at the aggregate results. Of course, care still needs to be taken to identify and compensate for systematic biases.
Granular modelling is reusable because it makes no assumptions about the segmentation approach adopted and the segmentation characteristics available. Modelling can be restricted to characteristics that are available in both source (already managed) and target (to be evaluated) portfolios.
The magic of granular, data-driven modelling is that it allows us to use past information to make predictions about very different portfolios. As long as we have enough past information about customers similar to the ones in the portfolio in question, the portfolio’s overall characteristics and the proportions of the segments do not matter.
The expense of granular modelling is fully mitigated by its potential for automation, and its ability to serve the needs of multiple stakeholders requiring different views or segmentations of the results.
For more information read our White Paper: Credit Portfolio Valuation: A Comprehensive Approach.
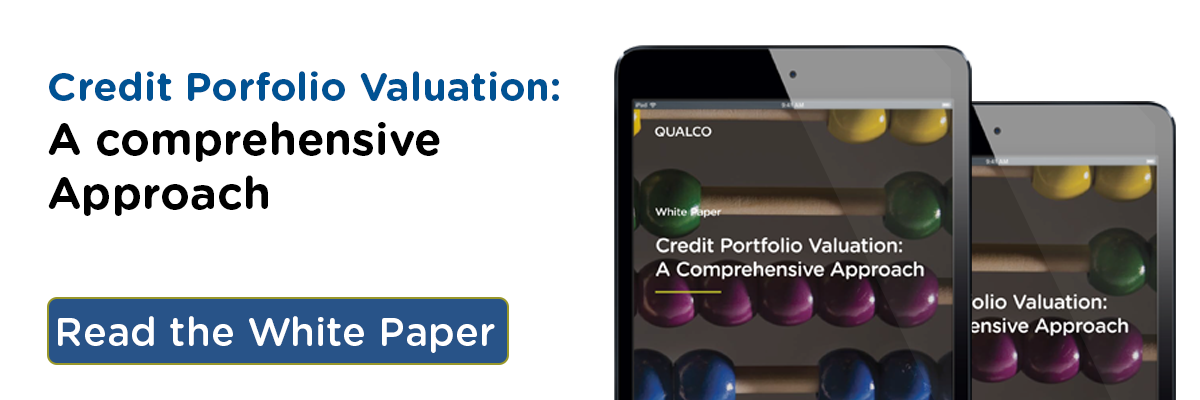