Contrasting and combining two approaches to portfolio valuation
Process modelling is concerned with the step-by-step processes by which a portfolio’s value is extracted. Data-driven modelling, on the other hand, relies on the use of past performance data – whether from the portfolio in question or other portfolios – and uses those data to make predictions.
So which approach is more useful? The answer is both. A valuation process needs to combine multiple approaches. Depending on the type of debt and the data available, a different mix of process and data-driven modelling comes into play.
Process Modelling
In cases where portfolio value is dependent on complex processes with little uncertainty and work without suitable past performance data, process modelling can be the optimal choice, as it enables value to be calculated for each step.
For example, when dealing with the liquidation of a secured portfolio, process modelling would seek to describe the legal process leading to liquidation and estimate timeframes, costs and failure probabilities at each stage of this process.
Data-Driven Modeling
When it comes to transferring information between portfolios of overlapping but differently proportioned components, data-driven modelling utilises the power of machine learning or statistical methods to identify and quantify drivers of customer behaviour.
Data-driven modelling assumes that our treatment approach for the new portfolio will be similar to that for the portfolios on which the model was trained but does not need to go into great detail regarding what that approach actually is. It is also resilient in the face of differences in the makeup of past and new portfolios, as long as the drivers can be assumed to be similar.
However, only a small subset of data-driven modelling methods can deal with driver variation within such portfolios, and all of them require a substantial amount of relevant past data to reach reliable conclusions.
Combining process and data
Once the core segments (guaranteed collectability, secured, unsecured, and limited collectability) are identified in a portfolio, pre-existing models can be selected and the data mapped. This data-driven modelling usually applies to segments that are unsecured or of limited collectability and are to be treated in a similar way to similar portfolios already under management.
Next, segments can be evaluated by data-driven modelling using new models built on data delivered with the portfolio. This approach applies to segments that are already under a type of management similar to that the organisation intends to apply. It may also be used as a baseline for estimating improvements to the management so far through process and empirical modelling.
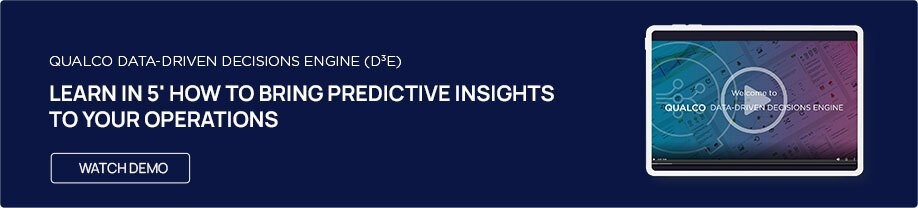
Other segments benefit more from the process modelling approach – such as secured or limited collectability segments whose value is driven mostly by statutory processes, rather than by their behavioural characteristics or the organisation’s treatment choices.
Secured segments expected to be unresponsive to collections processes and segments under legal (e.g. insolvency) protection, are typical examples, but simple types of process modelling may also apply in combining the results of data-driven models with process and capacity constraints.
Data-driven and process-based modelling help us adapt knowledge of the behaviour of past portfolios to deal with future ones, but we will inevitably come across cases where no suitable data are available: this is where empirical modelling comes into play, leveraging analyst experience to go beyond what the data have to say. Deciding which approach to employ for every portfolio and category within it is key to reaching a realistic valuation.
For more information read our White Paper: Credit Portfolio Valuation: A Comprehensive Approach. 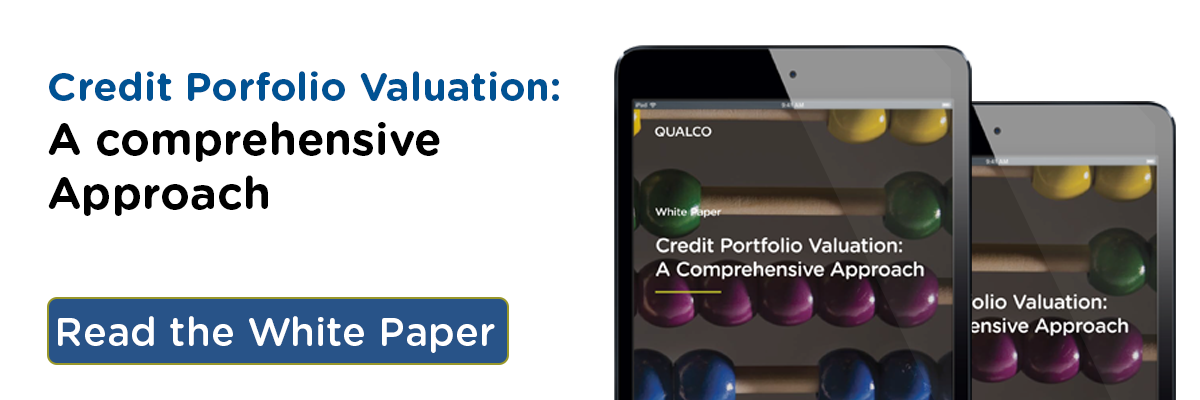